Online Road Complaints Registration System
Analysis on Complaint Behavior of Electric Power Customers
The data of electric power customer complaints from EZ power supply company in Hubei Province from January 2015 to May 2018 is obtained. During this time period, EZ power supply company received a total of 622 complaints from 95598 hotline. The annual distribution of complaints is shown in Table I. The highest number of complaints was in 2016, a total of 238, which fell sharply in 2017, but rebounded in 2018. There were 75 complaints from January to May 2018, with an increase of 62 cases compared with the number of complaints in the same period in 2017. The complaints were mainly concentrated in the rural network, a total of 507 cases, accounting for 81.51%. There were 115 cases in the urban network, accounting for 18.49%. Code Shoppy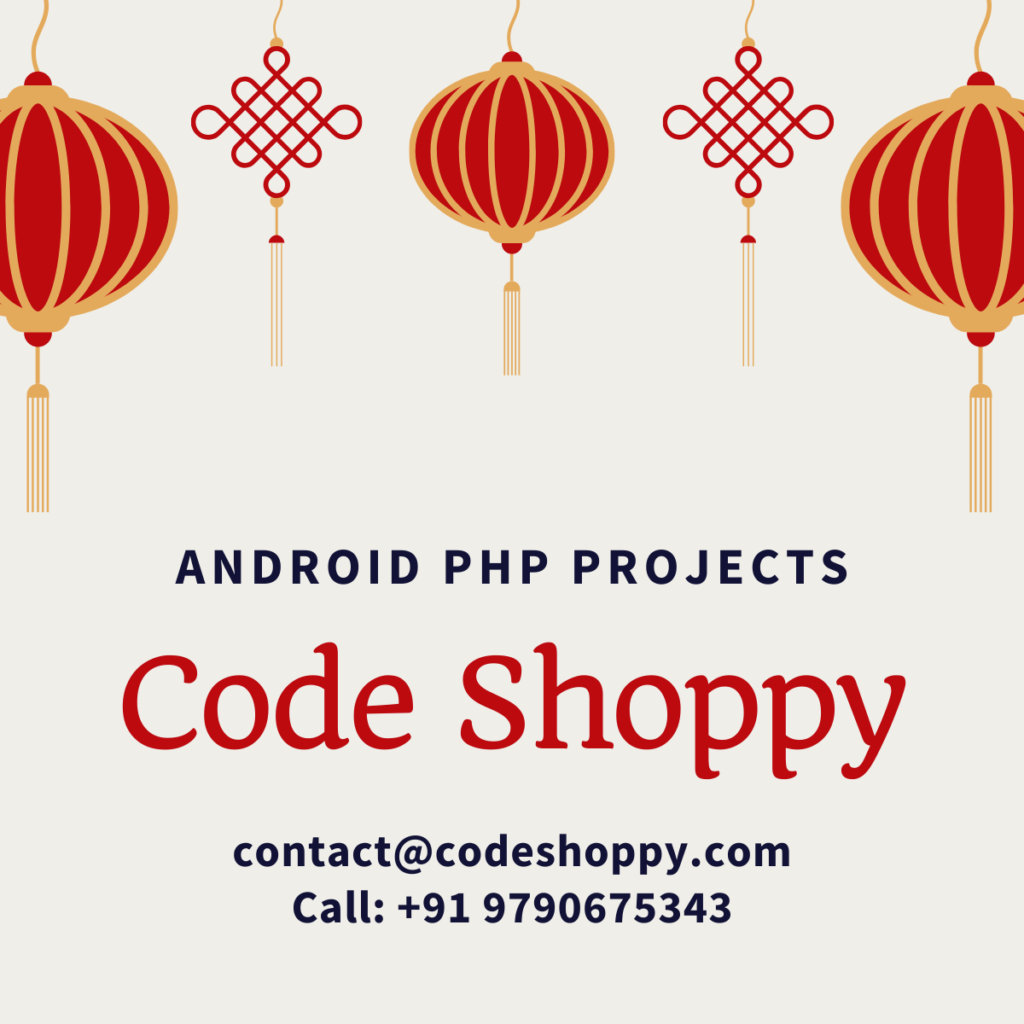
The number and proportion of customer complaints from 2015 to 2018 are shown in Fig. 1. The proportion of power supply quality complaints and power grid construction complaints increased at first and then decreased, which indicated that the effect of power network reform in the past three years was remarkable. The proportion of business complaints and power outage and supply complaints decreased at first and then increased.
There are 24 power supply stations in this regional power grid. Each power supply station is responsible for one town. The population of each town is different. Fig. 9 shows the total number of complaints and the number of complaints per 10,000 households in each power supply station. HH power supply station has the largest number of complaints among these 24 power supply stations, with a total of 64 complaints. The biggest amount of complaints per 10,000 households is YY power supply station, with 28.43 cases per 10,000 households.
From 2015 to 2017, complaints from seven power supply stations, such as HH power supply station, have declined year by year. There is no complaint from CG and JK power supply stations since 2017, and no complaint from DS power supply station since 2016. However, complaints from TH power supply station increase year by year. From January to May 2018, the total number of complaints from 19 power supply stations increase year-on-year. The complaints from GK power supply station have the maximum increase, with an increase of nine. There are 20 power supply stations whose power supply quality complaints have the largest proportion of the total number of their complaints. Fig. 10 shows the distribution of power supply quality complaints in power supply stations. GK power supply station has the largest number of complaints about power supply quality, with 32 cases. Four power supply stations have no power supply quality complaint for three consecutive years. The number of power supply quality complaints per 10,000 households in YY power supply station is the maximum, 16.72 cases per 10,000 households.
Fig. 11 shows the distribution of business complaints from power supply stations. HH power supply station has the largest number of business complaints, with 21 cases. Three power supply stations have no business complaint for three consecutive years. The number of business complaints per 10,000 households in YY power supply station is the maximum, 8.36 cases per 10,000 households.
Fig. 12 shows the distribution of service complaints from power supply stations. HH power supply station has the largest number of service complaints, with 10 cases. Four power supply stations have no service complaint for three consecutive years. The number of service complaints per 10,000 households in DD power supply station is the maximum, 4.01 cases per 10,000 households.
Fig. 13 shows the distribution of power grid construction complaints from power supply stations. TZH and ZS power supply stations have the largest number of power grid construction complaints, with 6 cases. Three power supply stations have no service complaint for three consecutive years. The number of power grid construction complaints per 10,000 households in TZ power supply station is the maximum, 5.63 cases per 10,000 households.
Fig. 14 shows the distribution of power outage and supply complaints from power supply stations. GD and HH power supply stations have the largest number of power outage and supply construction complaints, with 8 cases. Eight power supply stations have no power outage and supply complaint for three consecutive years. The number of power outage and supply complaints per 10,000 households in TH power supply station is the maximum, 3.27 cases per 10,000 households. Click Here